Introduction
Scientific research can be a great way to understand our world. Scientific exploration starts with careful, hopefully unbiased, observations. As Rolfers, we are observing our clients before and after our interventions. The outcome might be a visible structural change or some sensory adaptation, such as a reduction in pain or improved comfort. We may believe that the observed change is due to our intervention, but just because two events happen at the same time does not mean one caused the other to happen. We need our clinical observations to be confirmed through valid experimental studies before we can claim a relationship exists between our fascial interventions and the client change.
The first step is this observation of a single case, which can only be considered a description of variables at play. To find trends that generalize to the general population, many clients need to be observed empirically, with valid data collection methods. Data from many clients collected as a cross section of a single point in time or data from a subset of many subjects presenting with a specific characteristic (like lumbar pain) would give more power to claim a relationship between fascial intervention and client outcome. Then predictions can be made about client outcomes, experimental designs can be applied to the Ten Series, and specific hypothesis testing is possible. The scientific method applied to the work of structural integration (SI) can reveal specific interactions that happen in our offices every day. The purpose of this article is to empower Rolfers to be discerning consumers of empirical research, to discriminate how the design of a clinical study will have inherent strengths and weaknesses.
We know that not everything published is perfect or true. In fact, there are websites such as retractionwatch.com that spotlight bad research. Ivan Oransky, co-founder of Retraction Watch, estimates that of the two to three million articles published each year, 500-600 are retracted. While that is a relatively small number, it is still important and can have major implications. For a high-profile example, a study linking vaccines and autism that was published in The Lancet in 1998 was retracted in 2010 after it was found that the study design was seriously flawed (Wakefield et al. 1998).
Only a very small fraction of retracted publications are due to fraud. There are many other studies that don’t hold up to scrutiny either because they are poorly designed, are found not to be repeatable, or are published in ‘predatory journals’ that don’t really have good quality-control mechanisms, such as an expert peer-review system. Even when articles are peer reviewed, it is often difficult even for the experts to identify flaws in a study because of researcher bias, funding complexities, population unknowns, or the isolated nature of specialized research.
A contemporary limitation to published research is the ‘desk-drawer phenomenon’. The desk-drawer phenomenon applies to when the result does not show significant differences between treatment groups (research participants who received the experimental manipulation) and the control group (research participants who did not have any intervention), which is called a null result. The researchers often will not publish this data when this information may in fact be valuable, so it gets put away in the desk drawer even though the absence of a relationship between two variables is still important information.
Each type of study design applied by researchers has errors built into the system; although they are supposed to report these flaws in the paper, they can fail to mention any number of biases. For example, while a study may describe the results as an accurate reflection of the general population, the participants might have only come from a limited representation of the population – such as all participants were eighteen-year-old university students, all participants were upper-middle-class Caucasian men, or all participants lived in rural Arizona. It is important to look at who the participants were in any study because that will determine how much can be generalized about the results. Studies may be ‘under-powered’ such that the results are statistically invalid. When participants stop their involvement before the study is over, their data is usually discarded, and the dropout rate should be mentioned by the researcher. This is called the ‘discontinuation rate’. The reason people drop out during a study is significant, and their missing data points can skew study results. Everything pertaining to how that data is handled can greatly alter the study interpretation, especially the selection of participants.
Researcher bias may influence how data is evaluated and interpreted, and funding sources can have an unconscious influence on the researcher’s logic, so we should check to see what funding sources were used to execute the research. Corporate funding of studies is always concerning in that the study design itself can be prospectively influenced by the funding mechanism. National research boards have the best reliability for neutral funding streams, based on the merit of the academic and the topic. An independent researcher will often execute clinical studies for pharmaceutical corporations, with specific ‘firewalls’ set up between the research decisions and the corporation. Nevertheless, funding influence, or even the perception of influence, is hard to dispel.
Rolfers are constantly scanning their subjects to determine where an intervention will be most effective. This might be due to a static postural observation, an observed movement pattern, or some characteristic that a subject perceives such as pain, tightness, or other more abstracts feelings. It is then incumbent on us when we perform an intervention to observe the changes. This can be an informed observation based on the research we read. So, while an initial observation on one subject might be interesting, it lacks empirical strength. But if we find a peer-reviewed article about that variable of interest that has been evaluated with a large group of participants, we can use this evidence and apply it to our practice. Ideally, we would benefit from large studies with control group and treatment group comparisons of fascial interventions to understand the deeper context of what we see in our offices. For this reason, it is important that the SI community critically examine our observations and those contained in published data to ascertain that we use this information appropriately.
With all the information available on the Internet, publicly available databases, and self-publishing, we have without a doubt more information than ever before available at our fingertips. As Rolfers we have to sort through all this information and have a critical mind to find accurate information. Many of us read the title of a published article – or perhaps a newspaper article referencing a recent publication – and have the urge to post the findings to our peers without scrutinizing how the conclusions were reached. Is the data really supportive to that conclusion? Could there be stronger information on which to decide? Is there bias in the experimental design? Here are some reliable steps to thinking analytically about research, to help you weed out less reliable conclusions.
Why Peer-Reviewed Journals?
The first step to finding good research is seeking it in peer-reviewed journals. A peer-reviewed publication is, by definition, a journal that subjects each submitted article to evaluation by one or more knowledgeable individuals (peers). Typically, corrections and suggestions are given before these articles are refined enough for publication. Science relies heavily on critical peer reviews. While that doesn’t fully guarantee the quality of the study data, it does give public confidence that the experts put their reputations on the line when they state the information can be trusted as reliable. This evaluation process can be credited with screening out submitted manuscripts with serious design flaws, yet we also must have a critical eye when reading peer-reviewed studies because bias and error can still exist.
Empiricism and Scientific Inquiry
As Rolfers, or any person interested in how the world works, we need to develop our sensory experience of the world without pre-interpretation. This requires that we observe and reflect on sensory observations as pure information, without judgment, and we must put aside preconceived ideas dictating our understanding of the world around us. The same sensory information is available to all of us, and with normal sensory capacity, a group of people observing a specific phenomenon will share similar descriptions. This process of relying on sensory phenomenon to understand our world is the foundation of empiricism. It is this concept of empiricism, combined with scientific methodology, that gives the basis for our current understanding of the world.
In our Rolfing® SI practices, we will make observations about our clients, collect data about their life experience, and we are tempted to generalize from one client to all clients who present in a similar way. For instance, we may observe that someone has better balance after completing the Ten Series. To be thorough, we would need to observe many clients and compare them to people who did not receive any SI work. If we compare the variable of balance in subjects who have completed the Ten Series compared to the general public, we may indeed find that the SI subjects have better balance. This is termed a correlation approach, where one variable is observed with a control group (here it would be those who did not have the SI intervention) and an experimental group (here, it would be those who had the full Ten Series). It is important to note that a correlation does not, in itself, prove a causative relationship. A correlational study cannot claim that one variable caused the other to change since there may be other factors at work.
It is complex to determine a causative effect in clinical studies. If I do this specific fascial intervention, or a full Ten Series, will a predictable outcome be consistently observed? First, the method of observation must be valid and reliable. Like Dr. Rolf did with her photographic evidence, measuring angle of change in the vertical and horizontal orientation of the body can be one way of consistently evaluating change or no change. Experimental design requires strict controls of factors that are not being measured, like room conditions, time of day of intervention, trauma history, and socioeconomic factors to name a few. In a true experiment, participants should be randomly assigned to either the control group or the experimental group. This process where we influence a variable directly with a consistent intervention and compare that outcome with the group that had no intervention forms the basis of experimental methodology.
The past few years have been an exciting time for Rolfing SI research. There have been several publications assessing the therapeutic effect of SI for specific conditions, including cerebral palsy (Hansen et al. 2012; Hansen et al. 2014); fibromyalgia (Stal et al. 2015, Stal and Teixeria 2014); and, more recently, the first randomized trial to assess SI as adjunct therapy to outpatient rehabilitation for chronic non-specific low back pain (Jacobson et al. 2015). The Rolf Institute® of Structural Integration (RISI) website contains a listing of many peer-reviewed articles related to Rolfing SI on the Research page in the Visitors section.
Conceptualizing Clinical Research Design as a Hierarchy of Evidence
Considering that different study designs will yield different quality levels of evidence, with varying degrees of potential bias, let’s go through the varying strengths and weaknesses of common types of clinical studies. There has been significant discussion over the past twenty-five years regarding how to grade the different trial designs. Thus, many working groups have now published varying preferred hierarchies when evaluating clinical-trial designs since this concept was first introduced in 1992 (Guyatt et al. 1992). It should be noted that there is still debate about exact ordering of this hierarchy (Oxman 2004), and whether randomized controlled trials (RCTs) are truly superior to some observational study designs (Concato et al. 2000).
Nevertheless, I present here (see Figure 1) a research hierarchy for the types of clinical study designs to assist us as Rolfers to have an awareness and context of observational designs compared to experimental designs, which is a process where researchers start to develop hypotheses and then to test those hypotheses. The goal of most clinical research is to be able to reliably predict client outcomes. As you can see, the bottom layer of the research hierarchy pyramid is the initial uncontrolled, observational study design of reporting on a single case: one data point described with great detail. Next are cross-sectional studies, also a type of observational study with more participants. These observational designs describe trends between variables. This begins the process of generating hypotheses about the relationship between variables of interest.
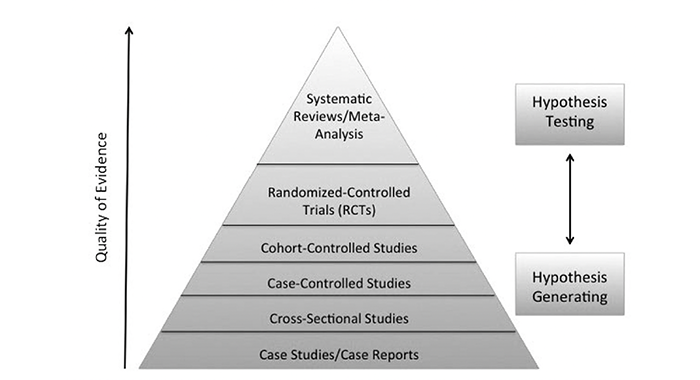
Once variable relationships have been replicated, researchers are then interested in testing their hypotheses. A case-controlled study is the first step in testing predictions. The researcher assembles a group of people who present with the variable of interest, such as suboccipital pain. The research assembles another group of people with similar demographics without the variable of interest. Data collection for both groups involves their past information, looking for the difference in their collective histories that might explain the symptom development.
Next, in cohort-controlled studies, the researcher assembles a large group of participants and does regular observations of their health over a long period of time. Some people in the group will develop the variable of interest, like chronic foot pain, and some will not. Then the researcher will look at what was different between the participants who developed symptoms and participants who did not.
Finally, randomized-controlled trials (RCTs) may include placebo treatment groups (participants who think they are getting the intervention but in actuality are not) and are considered by many to be the gold standard for clinical trial design (Concato et al. 2000; Stolberg et al. 2004). There is ongoing evaluation and discussion of the hierarchy of research design and its ordering (Oxman 2004). Above the RCTs in the hierarchy are systematic reviews or meta-analysis (Greenland and O’Rourke 2008; Walker et al. 2008). A meta-analysis combines data from multiple studies on the same topic to effectively increase the size of the database, yielding the strongest strength of the evidence.
The strength of clinical information improves as we move up the research hierarchy pyramid from initial observations on single cases to complex randomized controlled clinical studies and data sets involved in meta-analyses. The quality of the evidence starts broad, at the bottom layer, where a single phenomenon is described in significant detail. The evidence specificity becomes more refined as we move up the design pyramid. RCTs test predictions about the variables of interest and at the meta-analysis level there is a wealth of information where detailed theories can be described from the large data sets. All levels are useful to a researcher/practitioner. This is really the evolution of knowledge, discovered by the scientific method. It is important to differentiate the claims that can be made from each of these research designs since they do not all carry the same weight in their conclusions.
In our offices, it is very similar to the ground level of the pyramid, with initial observations on single subjects often being the starting point to researching new techniques or new applications. For instance, a client who presents with chronic plantar fasciitis and who receives three Rolfing sessions may be able to stand for longer periods of time and walk longer distances. Is this related to the Rolfing SI sessions or something else? While the observation is interesting, and in fact there may be a relationship, it is impossible to have any real predictability for future cases based on a single case study.
Studying an SI intervention in a RCT design might enroll sixty people or more with chronic plantar fasciitis, representatives of all ages of people, all heritages, all socioeconomic backgrounds, and equal number of males and females. Then the participants would be randomly assigned to either the treatment group, who will get the Rolfing sessions, or control group, who will get sessions of something unrelated. The best situation is when the group assignment is unknown to the researcher so the researcher is ‘blind’ to which participant is in which group. The researcher will analyze the data blind to group assignment, then un-blind as a last step to reveal true trends based on treatment. This removes researcher bias in the process.
Theory development happens as experimental research projects are published from several academic groups and when the collective results consistently converge to describe the same relationship between variables. These detailed discussions about causational relationships are often discussed in meta-analysis articles. The authors pool data sets from many studies looking at the same variables. This is the peak of the clinical research hierarchy. Even meta-analyses are not without their own inherent biases such as study data selection and the desk-drawer problem described earlier. So let’s go through each design type in detail so that a critical reader can read peer-reviewed publications and spot possible error or bias.
Case Study / Case Reports
Case studies and case reports are often used interchangeably, and generally refer to the study of a specific attribute of interest. The case may be one person or a group of participants with the same symptomology. Long histories are often reported about the case and there may be before and after an intervention described. The strength of this design is it often informs about interesting specific cases that might not be found in a larger study with many participants. It can also be the initial basis of interest for designing more complex studies. However, just because a change might be observed after a treatment, this cannot be generalized to every person who presents the same.
So, for instance, we may do a case study on a group of individuals and their response to Rolfing SI after hip replacement surgery. The study may examine a variety of variables, such as mobility, pain levels, recovery times, or other measures of well-being affected by the surgery. Writing case studies / case reports is already a part of the basic Rolfing training at RISI, and it is important to introduce students to the concepts of critically evaluating the intervention and the resultant observations. Reading more complex clinical research design is a part of putting our office observations into context.
Importantly, many adverse events are first reported in case studies, and these anecdotal observations are considered key initial steps leading to new scientific understanding. Case studies give us an avenue to share our own clinical experiences, and some clients are so unique that their particular process can give us unique understanding. Many of our Rolfing colleagues have already given us rich descriptions of cases, and there is an extensive listing of SI-related case studies/reports available online at The Ida P. Rolf Library of Structural Integration (www. pedroprado.com.br) maintained by Pedro Prado. Indeed, as SI practitioners, we are continually making case observations on our clients that often help us determine what interventions best integrate our clients.
Cross-Sectional Studies
Cross-sectional studies fall into the category of observational studies. The data is collected at one point in time, hence the term cross-section. The data is often used to describe risks within a given population. For instance, we might collect pain data on all subjects who have received Rolfing SI within the past year. This would give us a pain database – a descriptive slice, if you will, of the population of subjects recently having undergone Rolfing sessions. Cross-sectional studies use measures to turn variables into reliable numbers and provide statistical information about linear trends, such as prevalence information and certain risk measurements.
Cross-sectional studies are popular in peer-reviewed publications because they are the easiest to execute and the researcher only needs to interact with the participants once. This ease is its limitation since the data reflects the state of the participants at only one point in time. For instance, we often see clients who look better one year after completing a Ten Series. Just one meeting, one data point, is not enough to describe a person’s experience. The cross-sectional study is essentially a single snapshot in time. Sometimes the questions in the cross-sectional study are about things that happened in the participant’s past. This brings in recall bias, another limitation in observational studies. Recall bias reflects that subjects will have a selective memory for their past events, like all the times a person with neck pain had whiplash traumas. The compounding injuries are fresher in the person’s mind because of the neck pain, while someone without chronic neck pain may forget whiplash events more easily.
Case-Control Studies
Case-control studies are often used in epidemiological studies. It is a type of observational study in which the researcher looks at a large group of people who all share one type of symptomology. The researchers can compare them to an appropriate control group without the attribute and then look for what exposure differences between the two groups exist that might then be related to the condition. Hypothetically, one could look at subjects with plantar fasciitis and a control group of subjects without plantar fasciitis. One could then gather information on the two groups with regards to activities to find whether the prevalence of plantar fasciitis can be correlated with exposure to some earlier specific activity. Case-control studies are sometimes used to identify unknown factors, previously undescribed, that may contribute to a condition. Again, this is correlative information and just because variables vary together it does not say one variable is causing the other to change.
Cohort-Control Studies
A cohort is a group of subjects who have some shared characteristic or event within the context of some timeframe. For example, a cohort might be a group of subjects exposed to some risk factor, say playing football between the ages of fifteen and twenty-five. In cohort-controlled studies, a cohort is compared to a control group with similar demographics but not the same risk factors. The key aspect of this study design is that the groups are defined first and then data is collected over time as a longitudinal study. This helps to determine if there is a difference between the two groups in, say, chronic knee pain.
Cohort studies may also be prospective or retrospectively designed. In this example, a prospective design would look at subjects who played football and then follow them to determine if they develop chronic knee pain at a different rate than the control group. In a retrospective analysis, one cohort might have knee pain and then be asked whether they played football. The retrospective study design incorporates the possibility for recall bias. As with all study designs, cohort studies have the potential for bias, including the criteria for selection of the cohort and the control cohort.
Note that case-control studies will look at some known symptom incidence and then try to determine causal factors, whereas cohort-control studies look at an already suspected factor, such as football participation, and then determine the risk of developing the attribute (chronic knee pain).
Randomized Controlled Trials
RCTs are generally considered the gold standard in clinical-study design. The key strength is that the subjects are randomly assigned to a treatment group or a control group. Since the subjects are randomly assigned, this minimizes bias between subgroups, assuming the study is of sufficient size.
In this design, subjects are screened to see if they meet defined entry criteria relevant to the study design, such as age, ethnicity, medical condition, and background events. Once a group all with the same condition of interest has been assembled, they are then randomly assigned to one of the treatment or control groups. In a blind RCT study, the subjects are randomly assigned so that researchers are unaware of what treatment the participants are receiving when the data is being recorded. A double-blind study, mostly found in pharmacological research, is when the subjects are not aware whether they are receiving the active drug or an inert drug.
Despite being a strong research design, RCTs bias can still be introduced when the study is not of sufficient size or the randomization process resulted in different characteristics for the subgroups. There may also be different dropout rates between groups. Data can then be analyzed using the ‘as-treated’ analysis approach, which analyzes subjects based on the treatments they received. The data can also be analyzed using what’s termed ‘intent to treat’ analysis. Intent to treat analyzes all subjects based on their randomization, regardless of whether they received or adhered to the treatment plan.
It is complex designing an appropriate control treatment for a study evaluating SI. Often, complementary-health RCTs compare modalities with the same outcome measures. The clients will be randomly placed with various interventions as a comparison study, including what might be the current accepted ‘standard-of-care’ modality for the symptoms of interest. Randomized trials can also be designed as add-on designs to determine if adding a treatment, say Rolfing SI, to some other treatment modality will yield better results (Stal et al. 2015; Jacobson et al. 2015).
Meta-Analysis as a Systematic Review of RCTs
A meta-analysis is at the top of the study-design hierarchy pyramid along with RCTs. The meta-analysis combines and analyzes data from multiple RCTs to increase the data pool around a given treatment, intervention, or risk factor (Greenland and O’Rourke 2008; Walker et al. 2008). This improves the statistical power, maximizing the statistical predictability of treatment interventions causing change in measured variables and minimizing bias by the researchers wanting to see specific outcomes. As with any study design, bias may also be introduced in meta-analysis through bias in the original individual studies, or by which studies are selected to be included in the meta-analysis. The desk-drawer problem can make the meta-analysis data set incomplete. Since the studies with null results are often not published, some data is not included in the full picture of the phenomenon.
Cultivating a Critical Eye for Research Publications
Now that we know some basic differences in clinical-study designs we can understand that the outcomes from these studies can be conceived to fall in a hierarchy based on ‘strength of evidence’. To summarize, at the bottom of the hierarchy would be individual case studies, which have more limited predictive value. This information is characterized as low-strength evidence, or conversely, high bias. At the other end of the hierarchy are RCTs and meta-analysis, which are considered high-strength evidence with low bias.
Another way to characterize the progression in the hierarchy is often based on the order with which research data is generated. Initial research observations are typically from case reports or small clinical trials.
This is considered hypothesis-generating evidence, whereby the researcher sees some early observation and develops a hypothesis around that observation. Higher-level study designs, including RCTs, are then considered hypothesis-testing study designs in that their goal is to determine the validity of the hypothesis.
When you look at the conclusions of a published study, be sure you know what type of study design was employed. Also, make a note whether it was a peer-reviewed publication. Not that a peer review guarantees the results and conclusions, but at least it has gone through screening. By understanding the type of study design, where that falls on the hierarchy of evidence, and if the publication is peer-reviewed, the reader can better assess the validity of the information.
Bibliography
Concato, J., N. Shah, and R.I. Horwitz 2000. “Randomized, Controlled Trials, Observational Studies and the Hierarchy of Research Designs.” The New England Journal of Medicine 342(25):1887-1892.
Greenland, S. and K. O’Rourke 2008. “Meta-Analysis. Modern Epidemiology.” In K.J. Rothman, S. Greenland, T. Lash Modern Epidemiology, 3rd ed. Philadelphia: Wolters Kluwer Health/Lippincott Williams and Wilkins.
Guyatt, G., et al. 1992. “Evidence-Based Medicine: A New Approach to Teaching the Practice of Medicine.” The Journal of the American Medical Association 268(17):2420-2425.
Hansen, A.B., K.S. Price, and H.M. Feldman 2012. “Myofascial structural integration: A promising complementary therapy for young children with spastic cerebral palsy.” Journal of Evidence-Based Complementary & Alternative Medicine 17(2):131-135.
Hansen, A.B., et al. 2014. “Gait changes following myofascial integration (Rolfing) observed in two children with cerebral palsy.” Journal of Evidence-Based Complementary & Alternative Medicine 19(4):297-300.
Jacobson, E., et al. 2015. “Structural Integration as an adjunct to outpatient rehabilitation for chronic nonspecific low back pain: A randomized pilot clinical trial.” Journal of Evidence-Based Complementary and Alternative Medicine 2015:813418.Available at www.hindawi.com/journals/ecam/2015/813418/.
Oxman, A.D. 2004 “ Grading Quality of Evidence and Strength of Recommendations.” British Medical Journal 328(7454):1490.
Stal, P., et al. 2015. “Effects of structural integration Rolfing® method and acupuncture on fibromyalgia.” Rev Dor São Paulo 16(2):96-101.
Stal, P. and M.J. Teixeria 2014. “Fibromyalgia syndrome treated with the structural integration Rolfing® method.” Rev Dor São Paulo 15(4):248-252.
Stolberg, H.O., G. Norman, and I. Trop 2004. “Randomized Controlled Trials.” American Journal of Roentgenology 183(6):1539-1544.
Wakefield, A.J., et al. 1998. “RETRACTED: Ileal-lymphoid-nodular hyperplasia, non-specific colitis, and pervasive developmental disorder in children.” The Lancet 351(9103):637-41.
Walker, E., A.V. Hernandez, and M.W. Kattan 2008. “Meta-Analysis: Its Strengths and Limitations.” Cleveland Clinic Journal of Medicine 75(6):431-439.
Richard Ennis has a Bachelor of Science degree in ecology, ethology, and evolution, a Bachelor of Science degree in biochemistry, and a Master of Science degree in biology. He is a Certified Advanced Rolfer in Menlo Park, California and on Whidbey Island in Washington. Richard in on the RISI Research Committee, on the Scientific Advisory Committee for the Ida P. Rolf Research Foundation, and chairs the RISI Board of Directors.
Acknowledgements: Thanks to Cynthia Husted, PhD and Valerie Berg, Rolfing Instructor, for reviewing an earlier version of this manuscript. ■
View all articles: Articles home